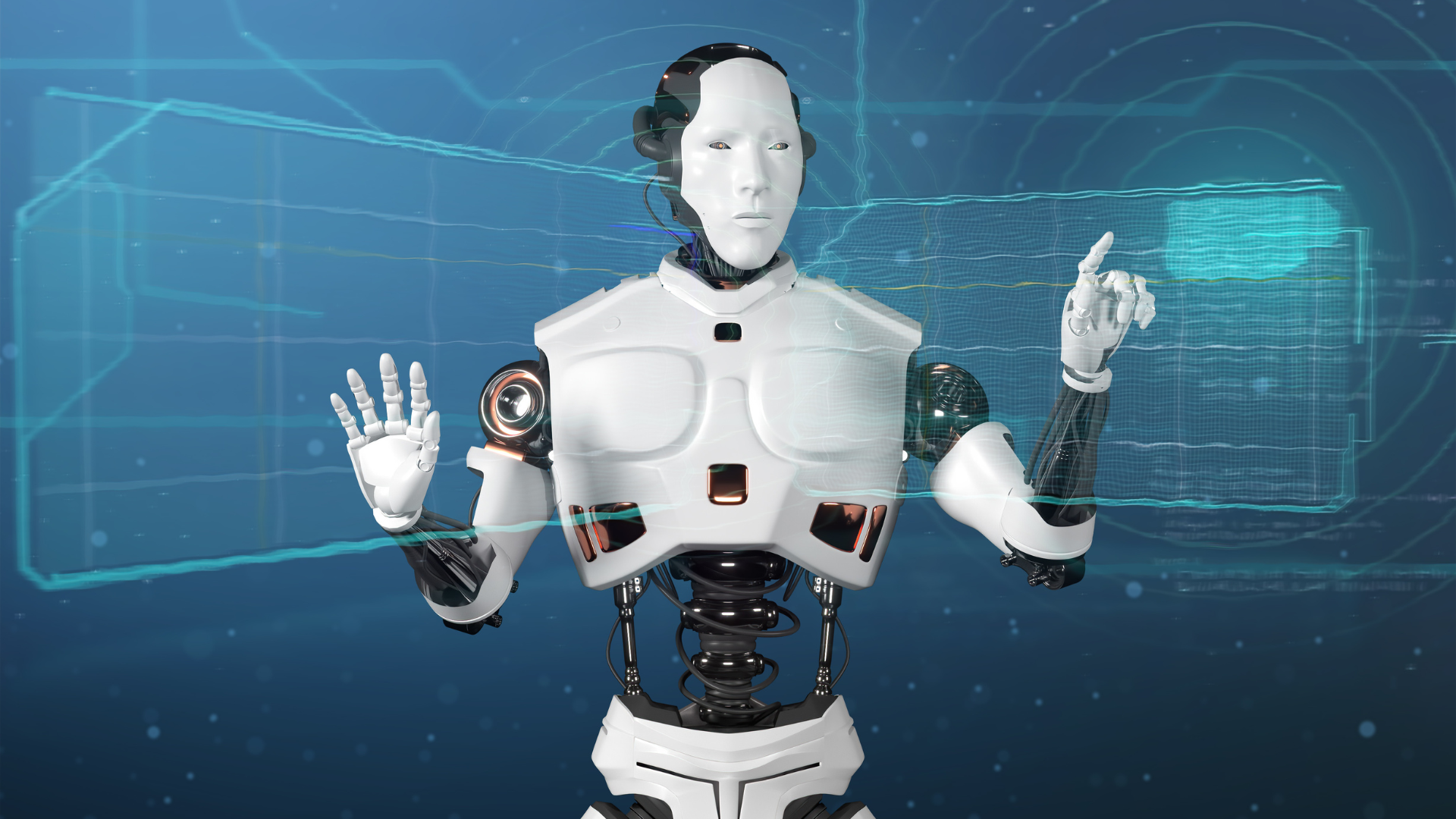
As artificial intelligence continues to advance and integrate into various aspects of daily life, the ethical implications surrounding its use demand critical attention. This includes considerations related to bias, accountability, privacy, and transparency. Addressing these ethical concerns is essential to ensure AI technologies are developed and implemented responsibly, fostering trust and safeguarding societal values.
AI poses unique challenges that must be managed thoughtfully. Issues such as data privacy and the potential for discriminatory practices highlight the importance of creating guidelines that protect individuals and communities while enabling innovation. Engaging in a dialogue about these topics is crucial, as it informs developers, policymakers, and users about the responsibilities associated with AI.
The conversation around ethical considerations in AI is not simply academic; it has real-world implications for everyone. As society increasingly relies on AI systems, understanding these ethical dimensions ensures that technology serves humanity positively and equitably. Attention to these issues encourages a future where technological advancements align with ethical standards and public interest.
Fundamentals of AI Ethics
AI ethics encompasses the guiding principles and considerations surrounding the development, deployment, and implications of artificial intelligence technologies. It examines how these technologies affect society, prioritizing fairness, accountability, and transparency.
Definition of AI Ethics
AI ethics refers to the moral guidelines and frameworks that govern the use of artificial intelligence. It seeks to address questions about how AI systems should be designed and implemented, ensuring they benefit humanity while minimizing harm. This includes evaluating the implications of automated decision-making and the potential for bias in AI algorithms. Ethical AI practices emphasize the responsible use of technology, ensuring that outcomes align with human values and social norms.
Historical Context
The concept of ethics in technology is not new. As computers evolved, ethical considerations emerged regarding privacy, security, and data ownership. With the advent of AI, these concerns intensified as machines began making decisions traditionally made by humans. Events such as the development of autonomous vehicles and facial recognition systems sparked debates over accountability and discrimination. Key historical moments, including MIT’s Media Lab’s 2016 study on algorithmic bias, highlighted the need for a robust ethical framework in AI development, prompting organizations and researchers to prioritize ethical considerations.
Key Principles
Several key principles form the foundation of AI ethics:
- Fairness: AI systems must be designed to avoid bias, ensuring equitable treatment across different populations.
- Transparency: Developers should maintain clarity in AI decision-making processes, enabling users to understand how outcomes are determined.
- Accountability: There must be mechanisms in place for holding individuals and organizations responsible for AI-related outcomes.
- Privacy: AI must respect individuals’ rights to privacy, ensuring proper data handling and security measures.
- Beneficence: The goal of AI should be to enhance human welfare and societal good.
These principles guide the ethical development of AI systems, promoting trust and responsible governance in their integration into society.
Ethical Frameworks in AI
Various ethical frameworks guide the development and implementation of AI technologies. Each framework offers a unique perspective on how to address moral dilemmas and responsibilities in the context of artificial intelligence.
Utilitarianism in AI
Utilitarianism focuses on maximizing overall happiness and minimizing suffering. In AI, this framework considers the consequences of actions taken by algorithms and systems. Decision-making algorithms should aim to produce the greatest good for the greatest number. For instance, AI in healthcare can prioritize treatments based on the potential for positive outcomes across a population. However, utilitarianism may undermine individual rights, as it could justify actions that harm a minority if they benefit the majority. Therefore, developers must balance outcomes with ethical considerations to ensure fairness.
Deontological Ethics
Deontological ethics emphasizes duties and rules regardless of consequences. This framework asserts that certain actions are inherently right or wrong. In the realm of AI, this means that developers must adhere to ethical codes and legal standards. For instance, an AI system processing personal data must follow privacy laws and respect user consent. Adopting deontological principles can prevent harmful practices, such as biased decision-making. This approach ensures accountability and promotes trust in AI technologies.
Virtue Ethics in AI
Virtue ethics centers on the character of the individual making decisions rather than the actions themselves. This framework encourages the cultivation of virtuous qualities like honesty, fairness, and compassion. In AI, a focus on virtue ethics emphasizes the importance of ethical engineers and developers. They must embody traits that promote responsible innovation and societal values. Virtue ethics can guide organizations to foster ethical cultures and decision-making processes. This can lead to AI technologies that reflect human values and enhance social well-being.
AI Accountability
AI accountability encompasses the mechanisms through which AI systems are governed, ensuring ethical usage and minimizing harm. Key dimensions include transparency, responsibility, and the processes for auditing and reporting.
Transparency
Transparency in AI refers to the clarity with which algorithms and their decision-making processes are presented. Organizations must disclose how AI systems function, including the data used for training and the criteria for decision-making.
- Data Sources: Clear documentation of the data sources helps users understand potential biases.
- Decision-Making Process: Outlining the logic behind algorithmic choices builds trust among stakeholders.
Transparency also involves user access to information on AI behaviors. This way, individuals can seek clarification or challenge decisions made by automated systems, fostering an environment of openness and trust.
Responsibility
AI responsibility focuses on assigning accountability for the actions and consequences of AI systems. It emphasizes that creators and operators of AI must ensure ethical standards are upheld during development and deployment.
- Clear Roles: Organizations should define roles and responsibilities for those involved in AI projects.
- Ethical Guidelines: Establishing and adhering to ethical guidelines ensures that AI is used responsibly.
By fostering a culture of responsibility, stakeholders can better handle issues that arise from AI implementation, such as discrimination or privacy violations, ensuring that ethical considerations remain at the forefront.
Auditing and Reporting
Auditing and reporting mechanisms are essential for evaluating AI systems’ performance and compliance with ethical standards. Regular audits ensure that systems are functioning as intended and adhering to regulatory requirements.
- Audit Frequency: Establishing a schedule for routine audits can help identify and rectify issues promptly.
- Reporting Findings: Transparent reporting of audit results to stakeholders promotes accountability.
This continuous evaluation process not only addresses current performance but also informs future improvements. Organizations can adjust practices based on findings, enhancing the ethical deployment of AI technologies.
Algorithmic Bias and Fairness
Algorithmic bias can significantly affect fairness in AI systems. Recognizing the sources of bias, the impact on various communities, and effective mitigation strategies is crucial for developing equitable AI solutions.
Sources of Bias
Bias in AI algorithms can arise from multiple sources. Data selection is a primary factor; if the training data is unrepresentative, the model may favor certain groups over others. Example sources include:
- Historical Bias: Existing societal inequalities reflected in data.
- Sampling Bias: Over- or under-representation of specific groups in the dataset.
- Labeling Bias: Human prejudices affecting how data points are categorized.
These biases can inadvertently become ingrained in algorithms, perpetuating inequalities previously present in the data.
Impact on Society
AI bias can lead to discriminatory practices that adversely affect marginalized communities. For instance, biased algorithms in hiring processes may overlook qualified candidates from certain demographics. Notable consequences include:
- Widening Inequality: Systems that disadvantage certain groups contribute to social disparities.
- Trust Erosion: Communities may lose faith in institutions employing biased AI systems.
- Legal Repercussions: Companies may face lawsuits for discriminatory practices tied to AI decisions.
The societal effects of algorithmic bias highlight the critical need for fairness in AI deployment.
Mitigation Strategies
Addressing algorithmic bias requires proactive strategies. Key approaches include:
- Diverse Data Collection: Ensuring datasets represent all demographics can mitigate bias at the outset.
- Bias Auditing: Regular evaluations of AI systems can identify and rectify biases.
- Algorithmic Transparency: Developing clear guidelines and documentation around algorithms helps stakeholders understand potential biases.
Employing these strategies fosters greater accountability and encourages equitable outcomes in AI applications.
Privacy and Data Governance
Privacy and data governance are critical components in the development and deployment of AI technologies. They encompass the management of personal data, ensuring ethical handling, and compliance with legal standards.
Data Protection
Data protection lays the foundation for safeguarding personal information in AI systems. Regulations like the General Data Protection Regulation (GDPR) mandate strict protocols for collecting, storing, and processing data. Organizations must implement measures to prevent data breaches and unauthorized access.
Encryption and anonymization are two essential strategies that can enhance data protection. By employing robust cybersecurity practices, companies can mitigate risks and comply with legal obligations. The importance of transparency in data usage also cannot be overstated, as it fosters trust between users and AI providers.
Consent and Ownership
Consent is crucial in establishing the parameters of data usage. Individuals must be informed about what data is collected and how it will be used. Clear and concise consent forms are necessary to facilitate understanding, allowing users to make informed choices.
Data ownership is another key issue. Users should retain rights over their personal data even once it is entered into an AI system. Companies often create terms of service that may obscure the ownership dynamics. Therefore, it is vital to clarify ownership rights and allow users to withdraw consent when desired.
Anonymity and Surveillance
Anonymity serves as a protective measure for individuals in the digital landscape. AI systems that prioritize user anonymity can reduce the risks of surveillance and data exploitation. Techniques such as differential privacy can help provide insights without compromising individual identities.
Conversely, surveillance raises ethical concerns related to privacy erosion. The continuous monitoring of user behavior can lead to a culture of mistrust. Organizations must balance the need for data to improve AI functionality against the imperative to protect users’ privacy. Transparent communication regarding surveillance practices is essential for maintaining ethical standards.
Human-Centered AI
Human-centered AI emphasizes the importance of prioritizing human needs and values in the development and deployment of artificial intelligence technologies. This approach aims to enhance collaboration between humans and AI, improve human capabilities, and ensure proper oversight.
Human-AI Collaboration
Effective human-AI collaboration requires designing systems that complement human skills rather than replace them. AI can support decision-making processes, increase efficiency, and reduce cognitive load. For instance, AI tools in healthcare can analyze data, enabling physicians to focus on patient care rather than administrative tasks.
Working in tandem enhances creativity and problem-solving. For example, AI can provide insights from vast datasets, which can help teams brainstorm more innovative solutions. This collaboration fosters a more productive environment and allows humans to leverage machine strengths while maintaining control and creativity.
Enhancing Human Capabilities
AI has the potential to significantly enhance human capabilities across various fields. In education, adaptive learning technologies cater to individual student needs, promoting personalized learning experiences. These systems assess student performance in real-time, adjusting content to maximize engagement and effectiveness.
In the workplace, AI can assist employees by automating repetitive tasks, allowing them to focus on higher-value activities. For example, AI-driven analytics can help professionals make data-informed decisions, leading to increased productivity and creativity. Consequently, the augmentation of human abilities can lead to more innovative outcomes and improved job satisfaction.
Human Oversight
Human oversight is crucial to ensure that AI applications align with ethical standards and societal values. Transparency in AI algorithms is necessary for stakeholders to understand the decision-making processes. This understanding helps mitigate risks associated with bias, discrimination, and accountability.
Implementing robust oversight mechanisms involves continuous monitoring and evaluation of AI systems. Regular audits can identify potential issues and improve system performance. Furthermore, involving diverse groups in the development process can ensure that multiple perspectives inform AI ethics, leading to fairer and more responsible AI applications.
Social and Economic Impacts
The integration of AI into various sectors brings significant social and economic changes. Examining its effects on employment, wealth distribution, and social inclusion highlights the complexities involved.
Employment and Labor Markets
AI technologies can enhance productivity but may also disrupt existing jobs. Various studies indicate that automation could replace routine tasks, leading to job displacement in sectors such as manufacturing and customer service. For instance, it is estimated that 20-30% of current jobs could be affected by automation by 2030.
On the other hand, AI also creates new job opportunities. Fields like data analysis, AI maintenance, and ethical oversight are expected to emerge. Reskilling workers will be crucial to minimize adverse impacts on the workforce.
Wealth Distribution
The adoption of AI may exacerbate wealth inequality. Companies that effectively implement AI can significantly reduce costs and increase profits, potentially leading to wealth consolidation. Wealthier organizations have the resources to invest in AI, while smaller firms may struggle to keep pace, widening the economic gap. Policymakers must consider strategies to ensure equitable access to AI technologies.
Social Inclusion
AI’s role in social inclusion is multi-faceted. It has the potential to improve access to services for underserved populations, such as healthcare and education. For example, AI-driven tools can enhance personalized learning experiences for students with diverse needs. Conversely, there are risks related to bias in AI systems. If models are trained on skewed data, they can perpetuate or amplify social inequalities. Addressing these biases is essential to ensure that AI benefits all members of society.
Global Considerations
As artificial intelligence continues to evolve, it raises ethical questions that require attention from a global perspective. Different cultures and nations have diverse views on ethics, leading to a complex landscape that needs careful navigation.
Cross-Cultural Ethics
Ethical standards in AI must accommodate cultural differences. Individualistic societies may prioritize user autonomy, while collectivist cultures might emphasize community welfare. For example, privacy concerns vary widely. In some cultures, data protection is paramount, while others may favor innovation over privacy. Understanding these perspectives is crucial for developing AI systems that are ethically sound across various regions. Engaging with local stakeholders can help identify specific ethical concerns that should guide AI deployment. This engagement fosters awareness and respect for differing values and norms in technology use.
International Collaboration
Collaboration among countries is essential for establishing global ethical guidelines in AI development. Various organizations and governments are beginning to work together to share best practices and formulate standards. Such collaboration can take the form of conferences, joint research initiatives, and policy dialogues. For instance, initiatives like the Global Partnership on Artificial Intelligence (GPAI) bring together stakeholders to explore ethical implications and develop shared principles. Coordinated efforts also address challenges like algorithmic bias and accountability. International cooperation can promote higher standards and establish frameworks that transcend local limitations.
Policy and Governance
Strong policy and governance frameworks are vital for regulating AI on a global scale. Countries must develop policies that reflect their unique contexts but also align with international standards. Legislation should cover issues like transparency, accountability, and fairness. For example, the European Union’s AI Act is designed to create a legal framework for trustworthy AI, influencing global practices. Countries also need to invest in regulatory bodies capable of overseeing AI technologies. Effective governance ensures adherence to ethical standards while accommodating the rapid pace of technological change.
AI in Practice
Implementing AI in various sectors necessitates a thorough understanding of ethical considerations. Industry standards, the ethical development of AI technologies, and relevant case studies illustrate how organizations navigate these complexities.
Industry Standards
Adhering to industry standards is crucial for ensuring ethical AI practices. Various organizations, such as IEEE and ISO, have established guidelines that emphasize transparency, accountability, and fairness.
Key Standards:
- IEEE P7000: Focuses on ethical considerations in system design.
- ISO/IEC JTC 1/SC 42: Provides a framework for AI standards.
These standards promote best practices and ensure compliance with legal regulations. Organizations that follow these guidelines can build trust with users and stakeholders while minimizing risks associated with AI deployment.
Ethics in AI Development
Ethics in AI development is imperative for creating systems that respect human rights. Critical issues include bias mitigation, privacy protection, and responsible data usage.
Important Considerations:
- Bias Mitigation: Developers must prioritize fairness and avoid perpetuating existing biases in datasets.
- Transparency: Clear communication about how AI algorithms function enhances user trust.
- Accountability: Organizations should be held accountable for the outcomes of their AI systems.
Developers are encouraged to implement ethical reviews at multiple stages of the development process, fostering a culture of responsibility and foresight.
Case Studies
Real-world examples provide insight into ethical AI practices. Companies like Google and Microsoft have faced scrutiny for their AI projects, prompting them to adopt stronger ethical frameworks.
Example Cases:
- Google’s AI Principles: A set of guidelines emphasizing fairness and safety in AI applications.
- Microsoft’s AI Governance: Establishing a dedicated team to oversee ethical AI implementation.
These case studies highlight both the challenges and successes organizations face while striving to implement ethical AI. Reviewing these instances helps other developers learn from established practices, fostering a broader understanding of responsible AI deployment.
Future Directions
As the field of AI progresses, it is crucial to address evolving ethical standards, anticipate future challenges, and forecast the long-term impacts of AI technologies. These aspects will shape the development and integration of AI within society and industry.
Evolving Ethical Standards
AI ethics is not static; it adapts as technologies advance. Organizations are increasingly recognizing the need for dynamic ethical frameworks. Existing guidelines require regular updates to remain relevant.
Key areas of focus include transparency, accountability, and fairness. Companies must establish clear protocols for decision-making processes. Such protocols ensure that users and stakeholders understand how AI systems operate and are held accountable for their results. Collaboration among industry experts, policymakers, and ethicists is essential. This collective approach fosters diverse perspectives that can inform and enrich ethical standards, promoting comprehensive AI governance.
Anticipating Future Challenges
AI introduces numerous challenges that necessitate proactive strategies. Issues such as data privacy, security, job displacement, and algorithmic bias demand attention. Stakeholders must identify risks before they escalate. Regulatory bodies play a pivotal role in establishing guidelines. Developing robust legislation can help mitigate issues arising from AI deployments. These regulations should adapt to emerging technologies, considering the fast-paced nature of AI advancements. Public engagement is also crucial. Involving communities in discussions about AI can help address misconceptions and highlight public concerns. This engagement promotes a more informed society that can navigate future AI developments responsibly.
Long-Term Impact Forecasting
Understanding the long-term impacts of AI is vital for effective policy-making. Forecasting potential outcomes helps assess the consequences of widespread AI adoption. This involves analyzing various scenarios, including economic, social, and ethical implications. Evaluating economic impacts includes job creation and displacement. It is important to identify sectors likely to thrive versus those at risk. Social implications extend to relationships, decision-making processes, and user trust in technology. Ethical considerations also extend to AI’s influence on societal norms. As AI becomes more integrated into daily life, it may reshape cultural attitudes toward privacy and human interaction. Assessing these long-term effects ensures that ethical considerations remain central to AI’s future.